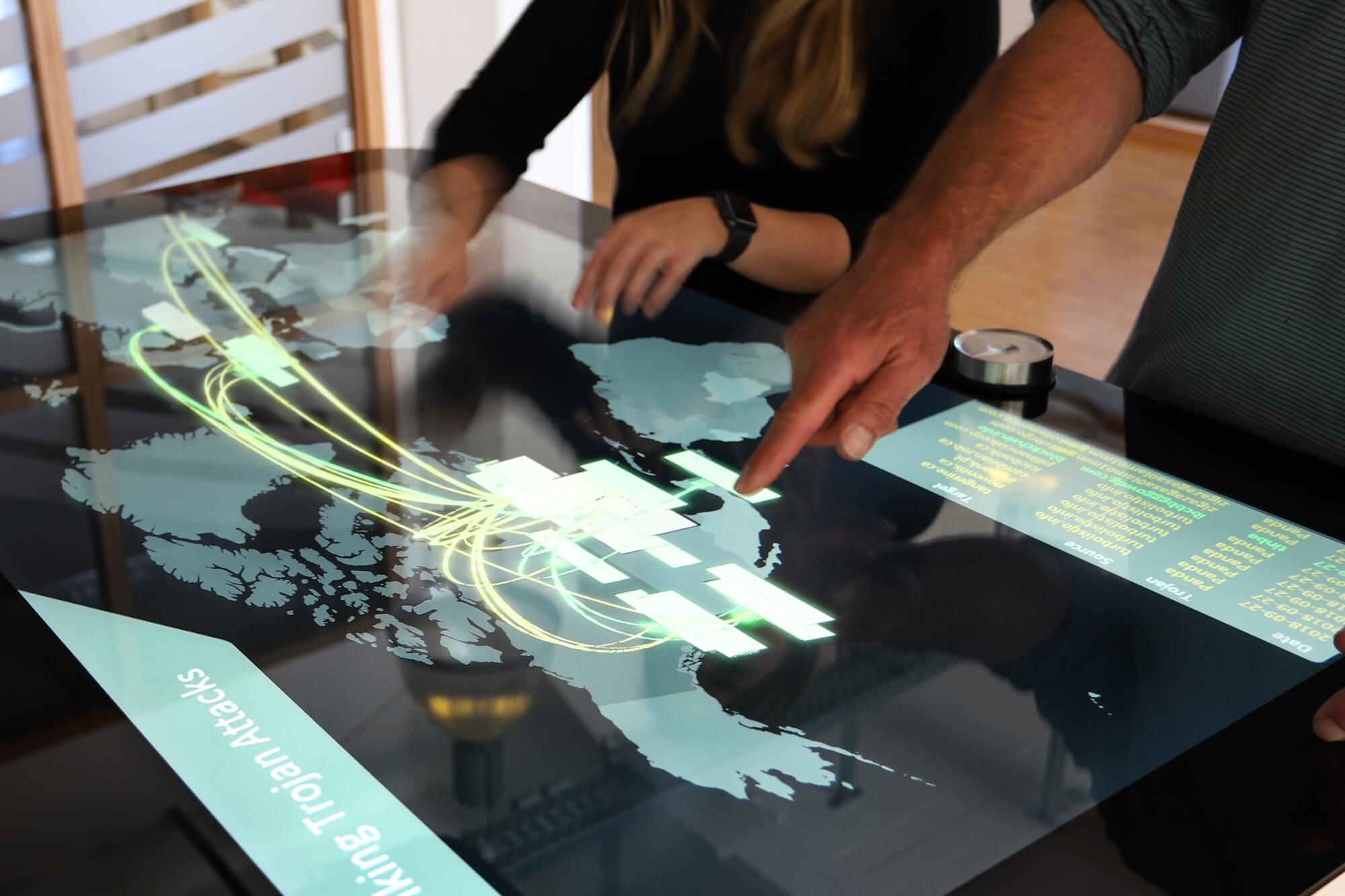
Virus Total is a reliable tool for all those who work with malware. Users can upload a file and check it for Malware. They can also try, if a files hash value is already in the database and if it is malicious or not. Journalists often use Virus Total, to check whether or not there is already effective protection against current malware campaigns by antivirus-solutions, or not. But due to next-generation technologies the answers are often misleading.
Especially in the case of very current and short-lived campaigns, the service often draws an inaccurate picture. At the beginning of the distribution of new malware, malware is detected by the antivirus programs of most security companies primarily through proactive detection technologies. Many AV-products also use the comparison of file hash values with cloud services of the respective manufacturers. However, Virus Total only maps the "classic" path of signature-based detection. In other words: "Virus Total is a good indicator for the detection of malware. However, the platform is not suitable to determine which malware is currently not detected," says Ralf Benzmüller, Executive Speaker of G DATA Security Labs.
In many cases some files are already detected as malicious, even when no signature-based detection is available. This is especially true for short-lived campaigns such as Ransomware-spam. "Thanks to our file cloud and other NGAV technologies, we can react very quickly to new malware campaigns. In some of the most recent cases, 11.6 percent of malware-detections have been based on our proactive technologies," says Thomas Siebert, Head of Protection Technologies at G DATA.
Antivirus software: More than just signatures for a long time now
The way antivirus works changed profoundly over the past 20 years. Criminals modify Malware-samples in ever faster bursts to avoid detection. Almost all manufacturers rely on next-generation antivirus components. Our file cloud can block suspicious samples much faster than classic signature updates. But the file cloud is only one part of our self-learning holistic system.
G DATA also developed the anti-ransomware technology. It reliably protects against unwanted hard disk encryption. For private customers this component is switched on by default, business customers need to activate the function manually. They are advised accordingly when setting up the G DATA software. The technology checks in the background to see whether suspicious commands such as mass encryption of files are triggered without an active user input. "Anti-Ransomware technology is often an early warning system for us," says Siebert. "If a previously unrecognized file performs malicious actions, we can request it for deeper analysis and use the information obtained to roll out broad protection for all users.”
Behavior-based detection of malware
The G DATA Behavior Blocker - the behavior-based defense against malware - is another next-generation technology. It blocks suspicious actions on the basis of certain pre-defined indicators.
This form of behavior-based malware detection detects, for example, when programs automatically create autostart entries or change other suspicious values in the Windows system database (registry). This is especially the case with file less malware. In addition, .exe or .dll files are detected that want to copy themselves into the system32 directory. Similarly suspicious is a change in the hosts files – which can relay requests to certain IP addresses or web pages to another address. This attack was used for attacks on online banking in the past. If some of these features are detected together, a recognition is triggered.
"To ensure recognition performance, several 100,000 samples are processed daily in the G DATA SecurityLabs. They are executed in a large cluster of automatic analysis systems ("sandboxes"). The features gained are stored in a huge graph-based database in the form of several million new nodes every day and connections between these nodes," says Siebert. This makes it possible to identify which characteristics in which combination and weighting should lead to recognition. Machine-learning methods are also used to support this process.
This is how we protect customers against yet-unknown threats.